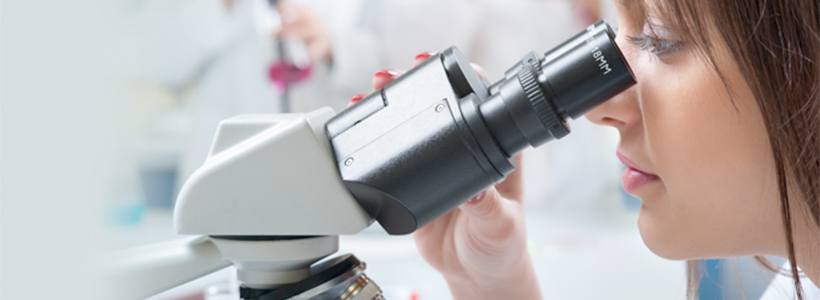
Moving object classification is a requirement in smart visual surveillance systems as it allows the system to know the kind of object in the scene and be able to recognize the actions the object can perform. This paper presents a neural-network machine learning approach for real time object classification in videos. This is necessary for the higher layer surveillance system to detect actions being performed by the moving objects. Fast kernel density estimation background subtraction algorithm is used for object segmentation. Radial distance signal features are then extracted from the silhouettes of the detected objects. The radial distance signals features are then normalized and fed into a multilayer feed-forward neural network to classify the object as human or vehicle. The neural network is trained using hybrid of genetic and back-propagation algorithm using features extracted from objects detected from real life video surveillance of human and vehicles and recognition was performed on some selected test data from the video objects. The recognition rate of 98.52% is achieved over back propagation algorithm and 99.13% with genetically trained neural network. is recorded. A comparison of this classifier with some other classifiers in terms of recognition accuracy shows a better performance than K-NN and K-Means classifiers while having the same recognition accuracy with support vector machines.