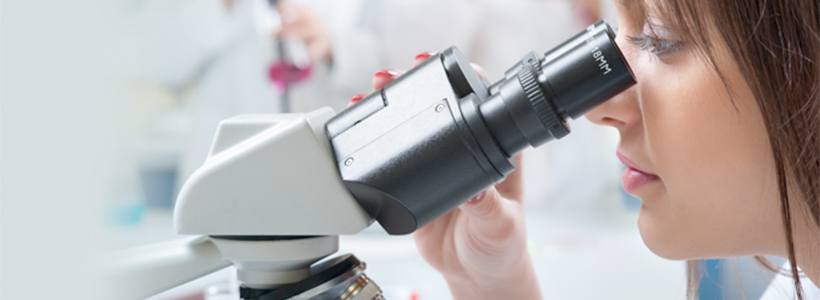
In surveillance scenario, some actions by human beings can generate alert actions. For example, a person jumping up and down in some environments may trigger alert action. There are some studies on hand gestures and sign language recognition. Automatic recognition of whole-body gestures is required in surveillance environment. This is a complex task in terms of segmenting meaningful gesture patterns from whole-body features. Few papers have been able to do this but the features used during computation is time consuming and may not be adequate in real time surveillance environments which require timely reporting of human actions. In this paper, effort is made to use light-weight features which are easy to extract and compute for whole body gesture action recognition in videos. To extract these features, silhouettes of actors are extracted from videos using background subtraction method. The features termed radial-signal distance features are then extracted from these silhouettes to form the feature vectors. The features are then quantized to obtain the code-words. A Left-Right Hidden-Markov-Model (LRHMM) is then constructed for each meaningful action. A threshold model is also constructed from the concatenation of all the states of the key actions Hidden-Markov-Model (HMM) models. The Forward algorithm and Viterbi decoding are then employed to spot and recognise actions patterns using the constructed models. Experiment performed on some video actions using the radial signal distance features shows a recognition accuracy of 93.16%.