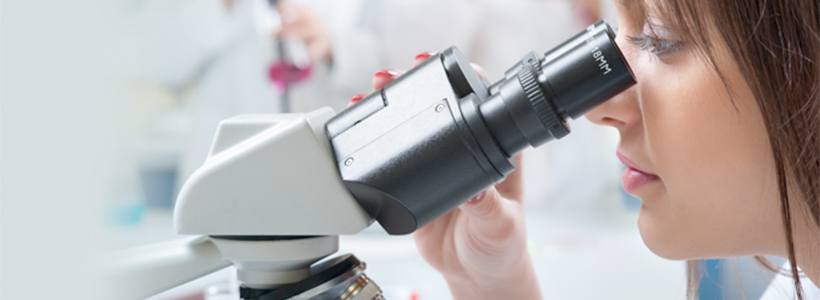
In recent years, shopping online is becoming more and more popular. When it need to decide whether to purchase a product or not on line, the opinions of others become important. It presents a great opportunity to share our viewpoints for various products purchase. However, data user faces the information overloading problem. How to mine valuable information from reviews to understand a product user’s preferences and make an accurate recommendation is crucial. Traditional recommender systems consider some factors, such as product user’s purchase records, product category, and geographic location we have seen a twist of review data processor sites. It displays an incredible chance to share our perspectives for different items we buy. In any case, we face the information overloading issue. Instructions to mine important data from reviews to understand a client’s preferences and make an exact recommendation are pivotal. Traditional recommender System (RS) think of some as elements, for example, client's buy records, item categorization, and geographic area. In this work, we suggest a sentiment based rating prediction technique (RPS) to enhance expectation precision in recommender frameworks. Firstly, we suggest a social client sentimental measurement proceed towards and compute every client's sentiment on items/products. Besides, we consider a client's own sentimental attributes but also take interpersonal sentimental influence into consideration. At that point, we consider item reputation, which can be induced by the sentimental distributions of a client set that reflects clients' complete assessment. Finally, we combine three factors client sentiment comparability, interpersonal sentimental influence, and item’s reputation similarity into our recommender system to make an accurate rating prediction.