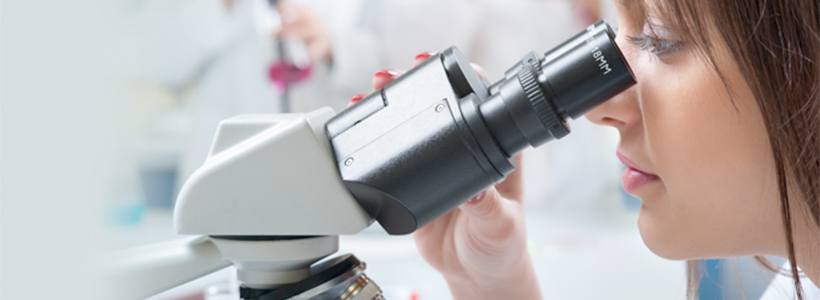
Diabetes mellitus (DM) is one of the global health challenge characterized by multifactorial pathogenesis and significant morbidity. Dyslipidemia, particularly the Triglyceride to HDL (TG:HDL) ratio, is increasingly recognized as a potential marker for diabetes risk. This study investigates the predictive potential of lipid profiles, focusing on the TG:HDL ratio, using advanced Machine learning techniques. A cross-sectional dataset of 1,000 participants was analysed, incorporating Logistic regression, Decision trees, Random forests, Support vector machines, and Gradient boosting models. Among these, Gradient boosting demonstrated the highest accuracy (88%), with the TG:HDL ratio emerging as a key predictor across all models. Exploratory data analysis revealed a strong correlation between elevated TG:HDL ratios and diabetes prevalence, supported by robust model performance metrics such as precision, recall, and AUC-ROC scores. The findings highlight the clinical utility of TG:HDL ratio as an accessible, cost-effective biomarker for early Diabetes detection and signifies the role of Machine learning in advancing personalized healthcare strategies.