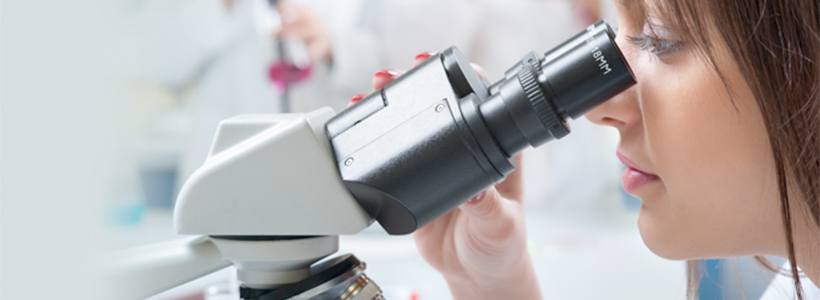
Urinary incontinence (UI) is a common condition that can severely impact the person’s lives. Machine learning (ML) techniques are recognized as a promising method for help in decision making. This review aimed to summarize existing ML models applied to any aspect of this affection.
A systematic search was performed in PubMed, Scopus and Web of science. Original studies targeting urinary incontinence were included.
The literature search identified 108 studies. 12 studies satisfied all the inclusion criteria and were retained. About75% of them were for prediction. The most used machine learning’s algorithm to develop the UI’s models were SVM in three cases. The area under the curve (AUC), was reported for six of the models, and their values were between 0.64 and 0.87. The accuracy was reported by five models and varied between 0.775 and 1. The sensitivity and specificity are reported for only three models. Their values were respectively (0.72; 0.675); (0.804; 0.774); and (0.996; 1).
In this systematic review, many models showed improved discrimination and accuracy. More research is needed to continue promoting this field of research in ML in order to obtain solutions with multicenter clinical applicability before these models can be incorporated into routine clinical practice.